Informatics
Disruptive precision medicine technology conquering inflammation and diabetes
Informatics Technology
The precision medicine informatics division at Biotherapeutics Inc has made it a priority to tackle the immensely complex challenge of discovering and validating new safer and more efficacious treatments for human diseases.
Problems with Current Drug Development Process
Developing a new medicine takes an average of 10-15 years. Of the tens of thousands of compounds screened, only one is approved.
How We Solve It
Our informatics technology platform is constructed and maintained by a team of outstanding immunologists, data scientists, computational modelers, bioinformaticians and software developers in order to address the comprehensive needs of precision medicine and conquer diseases with data.
Under our informatics umbrella, we incorporate a systems-wide analysis of massively interacting networks incurred between diet, microbiome, metabolism, immunity, pharmaceuticals and nutritionals. In doing so, the BTI team strongly invokes a holistic approach to successfully advance drug discovery in the precision medicine era.
iMed: intelligent Medicine
The advanced analytics platform, intelligent Medicine (iMED), is engineered to model from molecules and cells to clinical cohorts of patients with inflammatory bowel disease (IBD).
iMED is the first multiscale modeling platform that ties together Ordinary Differential Equation (ODE), Stochastic Differential Equation (SDE), Partial Differential Equation (PDE), Agent-Based Model (ABM), and Artificial Intelligence (AI) modules into a single cohesive IBD model capable of parallel processing.
iMed is able to elucidate hidden, unforeseen linkages between spatiotemporal simulation changes with clinical disease scoring methods through image analysis of endoscopies. Combined with expert image analysis of real patient’s gut endoscopic images, advanced machine learning algorithms provide a foundation for clinical utility of our iMED models.
EHR Data Mining and Synthetic IBD Patients
Using large-scale clinical data in the form of electronic health records (EHR) and claims data from insurance plans of IBD patients, BTI is developing synthetic IBD patient cohorts (i.e., human avatars that capture human diversity and variation) to perform in silico clinical trials and engineer personalized/precision medicine strategies tailoring individual patients. We use structured electronic health records of 11,325 IBD patients since 1996 to perform deep phenotyping through utilization of unsupervised machine learning methods. The data elements extracted include demographic information (including age, sex, race, age at diagnosis), laboratory measures (133 laboratory variables, including more common variables such as glucose level to less common variables such as cortisol levels in serum or plasma), medication history (medication name, dosage and timing), co-morbidities (complete list of ICD9/10 codes), as well as information from GI clinic visits. Using EHR, it is possible to build predictive models of diseases and response to treatments for unseen conditions. Integrating EHR data with other sources of clinical and preclinical data can provide valuable opportunities for knowledge discovery by providing venues for building models that can predict non-intuitive hypotheses.
AI involves advanced computer algorithms that replace the traditional rule-based strategy with data-driven approaches capable of learning from positive and negative experiences. As opposed to traditional “one-size-fits-all” treatments, AI algorithms are driving the future of predictive, preventive, precision medicine and provide personalized healthcare support for diverse and dynamic patient populations. On average, the EHR of a four-year patient contains about 32 petabytes of data. The application of AI will leverage the volume and exponential growth of clinical data to translate clinical information into new unforeseen insights for safer, more effective and cost-efficient personalized healthcare.
Synthetic patient cohorts are key components in the design of in silico clinical trials. A virtual population group ideally reflects the individual diversity and variability plus characteristics that represent a clinical cohort population. The virtual population can be extremely useful in identifying the broad range of responses which are otherwise hard to predict in a clinical trial.
A series of in silico clinical trials have been run based on de-identified clinical data of IBD cases. Data imputation was performed on the <250 samples of patient records. Machine learning (ML) methods were applied to predict INF-gamma, TNF-alpha, and CD Activity Index (CDAI) scores for the patients using profile information, lab measures, as well as medication history. A larger synthetic population of patient avatars was generated based on an expert guided random variation from the typical cases of IBD patients undergoing treatments of placebo, GED-0301, Anti-TNF-alpha, and BT-11. The EHR is used to create a training dataset for the prediction of CDAI scores of larger synthetic population of 10,000 patients using the optimized ML algorithm. From the different starting severity levels of CD, the statistically significant difference between the effects of the placebo versus all the drugs tested can be clearly seen (P < 0.05). For the highest range of initial CDAI scores from 450 to 500, BT-11 was statistically significant in outperforming GED-0301 and Anti-TNF-alpha in decreasing CDAI (P < 0.0001). These results suggest that BT-11 is the best drug option for treating the patients with moderate to severe IBD.
Personalized Nutrition
Around half of American adults have at least one chronic diseases, such as obesity and cardiovascular disease, which are often related to unhealthy diet and a sedentary lifestyle. Developing a healthy eating pattern in combination with an active lifestyle is vital to reduce the risk of disease and maintain health. Elevated blood glucose level is a major risk factor of diabetes. In a recent study, researchers from Weizmann Institute of Science measured responses (glucose levels) of 800 persons to 46,898 meals. It was found that the glucose levels can vary drastically in response to identical meals, indicating that the current, “one-size-fits-all”, dietary recommendations have limited value. Advanced analytics and big date related to nutrition, psychological sciences, and a growing demand from consumers for personalized products and services for improving human health lead to the need for personalized nutrition. We will leverage consumers’ data from food providers, distributors, retailers, smart devices, and healthcare provider to elucidate interactions among food/nutrition, human body (in particular human genome and intestinal microbiota), human behavior, and environment, based on which to prescribe food tailored to specific needs of the individual.
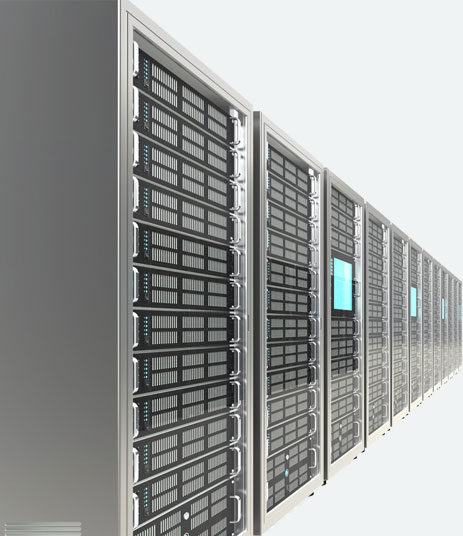